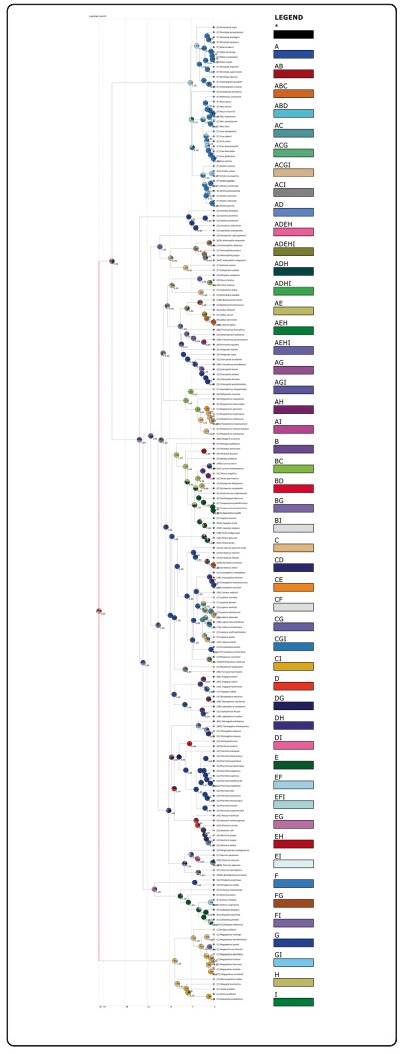
Citation: | Mengsi Wu, Yuchao Xiao, Fang Yang, Limeng Zhou, Weihong Zheng, Jinsong Liu. 2014: Seasonal variation in body mass and energy budget in Chinese bulbuls(pycnonotus sinensis). Avian Research, 5(1): 4. DOI: 10.1186/s40657-014-0004-8 |
Seasonal adjustments in body mass and energy budget are important for the survival of small birds in temperate zones. Seasonal changes in body mass, body temperature, gross energy intake (GEI), digestible energy intake (DEI), body fat content, as well as length and mass of the digestive tract, were measured in Chinese Bulbuls (Pycnonotus sinensis) caught in the wild at Wenzhou, China.
Body mass was determined with a Sartorius balance. The caloric contents of the dried food and feces were then determined using a oxygen bomb calorimeter. Total fat was extracted from the dried carcasses by ether extraction in a Soxhlet apparatus. The digestive tract of each bird was measured and weighed, and was then dried to a constant mass.
Body mass showed a significant seasonal variation and was higher in spring and winter than in summer and autumn. Body fat was higher in winter than in other seasons. GEI and DEI were significantly higher in winter. The length and mass of the digestive tract were greatest in winter and the magnitude of both these parameters was positively correlated with body mass, GEI and DEI. Small passerines typically have higher daily energy expenditure in winter, necessitating increased food consumption.
This general observation is consistent with the observed winter increase in gut volume and body mass in Chinese Bulbuls. These results suggest that Chinese Bulbuls adjust to winter conditions by increasing their body mass, body fat, GEI, DEI and digestive tract size.
Galliformes are ground-living birds, an order consisting of around 280 species worldwide (Chen, 2014). This group of birds is found to have around 23 species listed as endangered and 6 as critically endangered in the IUCN red list (The World Conservation Union 2010). Phylogenetic relationships among species have been widely studied in recent years (Crowe et al., 2006; Frank-Hoeflich et al., 2007; Hugall and Stuart-Fox, 2012). Although current phylogenetic information is still limited and does not cover the systematics and affinities of all 280 Galliformes, a recent investigation has established a phylogeny of Galliformes for up to 197 species (Hugall and Stuart-Fox, 2012), accounting for over 70% of global Galliformes.
Macro-evolutionary patterns of this large-size and attractive-appearance bird assemblage might be initiated by utilizing the available phylogenetic affinity information of the 197 species (Hugall and Stuart-Fox, 2012). The proposal to study macro-evolutionary patterns of Galliformes is to reveal in a more comprehensive way the extinction mechanism of this species assemblage from a long-term evolutionary perspective, for the purpose of better conserving them. In the present study, several macro-evolutionary attributes relevant to the phylogeny and diversity of Galliformes are considered.
In first instance, clade age has been thought to link up with species richness. The relationship between clade age and species richness is one of recent interest in macro-evolutionary studies (Rabosky et al., 2012; Rabosky and Adams, 2012). Clade age has been thought to relate to species richness because of the fact that older clades could have more time to diversify (McPeek and Brown, 2007; Etienne et al., 2012), implying that the older the age of the clade, the higher its richness, resulting in a positive clade age-species richness relationship. However, whether such a relationship is universal is still controversial. Several previous studies have shown that clade age could predict species richness (McPeek and Brown, 2007; Etienne et al., 2012), while others argued that there is no clear relationship between clade age and species diversity (Rabosky et al., 2012). In the present study, I wanted to test whether there is a relationship between the evolutionary age of the ancestors of Galliformes and the number of their externally living descendants.
Secondly, a shifting pattern in the rate of diversification has been broadly observed in many taxa, by showing patterns in which this rate is initially high but decreases over time (Rabosky and Lovette, 2008a, 2008b). Such a declining-diversification model is predicted by adaptive evolution (Schluter, 2000), because openings of new vacant niches are limited. Filling vacant niches would have been accomplished at an early evolutionary time, leading to a decline in the rate of diversification of species (Rabosky and Lovette, 2008b).
Finally, the size of their range might be related to the rate of diversification of species (Chen, 2013), since range size of a species is a trait jointly affected by species dispersal, colonization and reproduction. The importance of geographic isolation in shaping speciation has been debated for a long time (Fitzpatrick and Turelli, 2006). A phylogenetic comparative method, referred to as "age-range correlation", is introduced to quantify the relative importance of sympatric and allopatric speciation on structuring contemporary species diversity patterns (Fitzpatrick and Turelli, 2006; Bolnick and Fitzpatrick, 2007).
Considerable progress has been made in the development of robust statistical methods to infer ancestral ranges by incorporating a variety of biological processes. For example, a dispersal-extinction-cladogenesis model (DEC) has been proposed to estimate explicitly and infer historical changing patterns of ancestral ranges of species when projected on the phylogeny under a maximum likelihood framework (Ree et al., 2005; Ree and Smith, 2008). In addition to maximum likelihood-based methods for reconstructing ancestral distributional ranges, traditional methods are derived from the parsimony principle. Dispersal-vicariance analysis (DIVA) (Ronquist, 1997) and some of its extensions (for example, statistical DIVA (S-DIVA), Yu et al., 2010) are built on parsimony algorithms and still widely cited in current literature of phylogeographic studies (Ali et al., 2012). Recently, a Markov Chain Monte Carlo (MCMC) method has been proposed by Yu et al. (2013).
The ancestral ranges of the Galliformes group were estimated by utilizing the well-established phylogenetic tree for 197 Galliformes (Hugall and Stuart-Fox, 2012). The following terrestrial regions are considered in biogeographical analyses: East Asia (A), South Asia (B), Southeast Asia (C), West Asia (D), North America (E), South America (F), Africa (G), Europe (H) and Oceania (I). Distribution of each species over these terrestrial regions was obtained from the Avibase database (http://avibase.bsc-eoc.org/avibase.jsp?lang=EN).
In comparing ancestral ranges of Galliformes species, three analytical methods are used, i.e., dispersal-vicariance analysis (DIVA), Bayesian binary MCMC analysis (BBM) and dispersal-extinction-cladogenesis analysis (DEC). All three methods are carried out by using the software RASP (Yu et al., 2010, 2011).
Different diversification rate-shifting models have been tried to fit the Galliformes phylogeny, as proposed in previous studies (Rabosky, 2006b; Rabosky and Lovette, 2008a, 2008b). Specifically, four methods from an R package "laser" (Rabosky, 2006a) are implemented for comparative purposes, consisting of a constant-speciation and constant-extinction model (CONSTANT), a decreasing-speciation and constant-extinction model (SPVAR), a constant-speciation and increasing-extinction model (EXVAR) and a decreasing-speciation and increasing-extinction model (BOTHVAR) (Chen, 2013). These four models have been used to test the temporal shifting patterns in rates of diversification of different taxa (Rabosky and Lovette, 2008a, 2008b).
Each of the models require four parameters for estimation (Rabosky and Lovette, 2008b), which can be obtained by maximizing the following likelihood equation (Rabosky, 2006b):
L(t|λ(t),μ(t))=N−1∏n=2n(λ(t)−μ(t))exp{−n(λ(t)−μ(t))(tn−tn+1)}×{1−μ(t)λ(t)exp(−(λ(t)−μ(t))tn+1)}n−1{1−μ(t)λ(t)exp(−(λ(t)−μ(t))tn)}n |
(1) |
where t is the vector of observed branch times from the phylogeny, tn the branch time for the lineage, while n, λ(t) and μ(t) are time-dependent speciation and extinction rates, respectively. The time-dependent rate of diversification is defined as r(t)=λ(t)-μ(t) and N is the number of external tips in the tree.
In the CONSTANT model, λ(t) and μ(t) are assumed to be constant over the entire phylogenetic tree (i.e., λ(t) = λ0, μ(t) = μ0), where λ0 and μ0 are the constants to be estimated. In the SPVAR model, μ(t) is assumed to be constant over the entire tree (i.e., μ(t) = μ0), while λ(t) is assumed to decrease continuously from the root to the tips of the tree, defined as follows: λ(t)=λ0 exp(-kt). As seen in the SPVAR model, the additional parameter k is required to model the declining trend of rate of speciation over the tree. For this model, the rate of diversification is predicted to decline over the evolutionary time as (r(t)=λ0 exp(-kt)-μ0). In the EXVAR model, the rate of speciation is assumed to be constant over the tree while the rate of extinction is assumed to decline over the tree as follows: u(t)=u0(1-exp(-zt)). As well, this model has an additional parameter, i.e., z, to be estimated. Finally, in the BOTHVAR model, both λ(t) and μ(t) are assumed to change over time as follows: λ(t)=λ0 exp(-kt) and u(t)=u0(1-exp(-zt)) (Rabosky, 2006b; Rabosky and Lovette, 2008b).
The evolutionary age for each clade is calculated as the phylogenetic distance between the root and the internal node leading to the focused clade. The corresponding clade species richness is defined as the number of external tips (living species) for that specific clade.
To reveal the possible relationship between clade age and clade species richness and/or phylogenetic diversity, I performed both a non-phylogenetic ordinary least-squares regression analysis (OLS) and a phylogenetic general least-squares regression analysis (PGLS). Given that various clades are not independent from each other, it is necessary to remove the impacts of phylogenetic inertia by performing PGLS, i.e., a method to introduce a phylogenetic variance-covariance matrix in the fitting formula, a matrix missing in the OLS method. For the OLS method, the vector of coefficients is fitted using the following identity:
ˆβOLS=(XTX)−1XTy |
(2) |
while for the PGLS method, the vector of coefficients is estimated from the following equation:
ˆβPGLS=(XTW−1X)−1XTW−1y |
(3) |
The superscript T denotes the transpose of a matrix, while -1 denotes the inverse of a matrix. X is a matrix with columns indicating the explanatory variables, while y is a column vector storing the values for the response variable and W is the phylogenetic variance-covariance matrix. The calculation of W is only related to the branch lengths of the phylogenetic tree (Revell, 2010).
Both the Bayesian and maximum likelihood methods (BBI and Lagrange) identified SE Asia as the most likely origin of the distribution of the most common ancestor for all Galliformes species. The S-DIVA method failed to run because of unknown errors (out of memory when using RASP software).
Because BBI and Lagrange share some levels of similarity, ancestral ranges estimated by Lagrange are the focus in the subsequent biogoegraphic discussion (Figure 1). As seen, a number of dispersal and vicariance events have occurred in the distribution of Galliformes species.
At root node 393 (Figure 1), the most likely ancestral range is SE Asia and N America with a marginal probability of 29% (light orange color, symbol: CE). One vicariance event is identified, in which the Galliformes lineages in N America and SE Asia are separated in subsequent evolutionary times.
For the lineages distributed in N America, in later times at node 378 (Figure 1), two dispersal events occurred. Starting from N America, one ancestral lineage dispersed to S America (node 233, Figure 1, light blue color, symbol: EF) while another dispersed to Africa (node 377, Figure 1, purple color, symbol: EG). Again some time later (node 366), some lineages dispersed to E Asia (blue color, symbol: A).
For the lineage distributed in SE Asia at the root (Figure 1), it continued to inhabit that region and later underwent local radiation up until our contemporary era. During some evolutionary time points at nodes 391 and 389, some ancestral lineages of Galliformes dispersed to Oceania (green color, symbol: Ⅰ), leading to the contemporary distribution of Alectura lathami and Leipoa ocellata in Australia, Megapodius pritchardii in Tonga and Megapodius layardi in Vanuatu.
No significant diversification rate-shifting pattern is evident in Galliformes phylogeny. As shown by the comparison of different diversification models, the constant-rate model received the lowest AIC value (-621.62, Table 1) and thus became the best model.
Model | CONSTANT | SPVAR | EXVAR | BOTHVAR |
Likelihood | 312.81 | 312.69 | 312.81 | 312.63 |
AIC | -621.62 | -619.38 | -619.61 | -617.27 |
Parameters | ||||
λ0 | 0.174 | 0.193 | 0.1 85 | 0.186 |
μ0 | 0.064 | 0.014 | 0.011 | 0.001 |
K | - | 0.001 | - | 0.001 |
Z | - | 1.003 | 0.078 | |
The best-fitted model is marked in boldface. |
There is a significant and positive relationship between clade age and Galliformes species richness (Figure 2), regardless of whether the situation or whether phylogenetic inertia is controlled or not. For the OLS, the fitted equation of clade richness=1.24×clade age-8.29 (p < 0.05), while for the PGLS, the best fitted equation of clade richness=3.111 × clade age-101.48 (p < 0.05). This supports the prediction that older clades process higher species diversity since they have more time to diversify.
Interestingly, no significant diversification-shifting trend has been observed for the phylogeny of the 197 Galliformes species (Table 1). Several previous studies working on other avian taxa suggest a temporally diversification rate-declining pattern, for example that of the North American Wood-warblers (Rabosky and Lovette, 2008a). However, other studies also show that rates of diversification could have shown a temporally increasing trend up to our contemporary era for some specific species assemblages, for example Tiger Beetles (Barraclough and Vogler, 2002) and angiosperm plants (Magallon and Castillo, 2008). It is still controversial whether the rate of species diversification has a density-dependent trend throughout evolutionary times. From my observation of the Galliformes phylogeny, I conclude that this avian assemblage has a relatively constant rate of diversification over time, contradicting any time-dependent shifting trends of diversification.
There is a strong correlation between clade age and Galliformes richness as shown in Figure 2. As such, the current study confirms that clade age predicts Galliformes diversity throughout evolutionary times, but not rates of diversification. This conclusion is consistent with those of several previous studies (McPeek and Brown, 2007; Etienne et al., 2012). However, other studies have argued that there is no clear positive relationship between clade age and species richness (Rabosky et al., 2012; Rabosky, 2009) due to variation in rates of diversification among clades (Rabosky et al., 2007). Because I found that rates of diversification tend to be constant over evolutionary times for this Galliformes assemblage (Table 1), species richness of this avian group is principally driven by clade age (Figure 2).
As evidenced by the results of the Lagrange maximum likelihood analysis, it is found that SE Asia and N America are two disjunctive ancestral distributional origins for the earliest ancestor of Galliformes species. Dispersal frequency is very high for ancestral lineages of Galliformes. At some point in time, the lineage distributed in SE Asia then dispersed over Oceania while another lineage from N America dispersed to S America, Africa, E Asia and Europe. Active dispersals of Galliformes ancestors over the various continents might be an important driver of species diversity, because new vacant niches were available in these new terrestrial regions. Therefore, instead of rates of diversification, ecological opportunity might have played, implicitly, a role in species richness of Galliformes (Rabosky, 2009; Mahler et al., 2010; Setiadi et al., 2011).
Some limitations apply to this study. First, the phylogeny used contains only 197 Galliformes species, which might be not sufficient to unravel the true macro-evolutionary pattern of the assemblage because the remaining 83 species have not been included in this analysis. It has been suggested that estimating rates of diversification is very sensitive to the complete status of the tree, because incomplete taxonomic sampling could generate artificial diversification rate-shifting patterns (Cusimano and Renner, 2010; Brock et al., 2011). Second, estimation of the ancestral range is widely applied in plant biogeographical studies. Whether it is legitimate to infer historically ancestral ranges for bird taxa using plant-tailored statistical methods requires further elaboration. However, there is a growing trend in inferring ancestral life-history states of avian groups (Winger et al., 2012). To a certain extent therefore, it should be rational to estimate the ancestral distribution of Galliformes using the analytical DEC, S-DIVA and BBM methods. Third, utilization of country-level distributional information might not be sufficient to quantify range-clade relationships, given that country-level distributional records do not accurately reflect the true distributional ranges of Galliformes species. For example, some species might be present in a small area of a large country, leading to the over-representation of the distribution of the species.
In implementing further studies, the correlation of life-history traits and rates of diversification would be of interest, since it is reported that the ability of migration of avian species might slow down the rate of speciation of taxa (Ikeda et al., 2012; Claramunt et al., 2012). Analyses of the correlation between functional traits and rates of diversification would offer some insights into the relationship between dispersal capability and speciation patterns for Galliformes (Claramunt et al., 2012).
The constant diversification rate for global Galliforme species implied that there were no diversification rate-shifting trends for Galliformes species. The present study may contribute to the understanding of the ecology and diversity patterns of Galliformes from the perspective of historical biogeography, although some limitations existed.
The author declares that he has no competing interests.
This work is supported by the China Scholarship Council (CSC). I like to thank two anonymous reviewers for their insightful comments to improve the quality of the present work.
Biebach H (1996) Energetics of Winter and Migratory Fattening. In: Carey C (ed) Avian Energetics and Nutritional Ecology. Chapman and Hall, New York, pp 280–323
|
Karasov WH (1990) Digestion in birds: chemical and physiological determinants and ecological implications. Stud Avian Biol 13:39l–415l
|
Karasov WH (1996) Digestive Plasticity in Avian Energetics and Feeding Ecology. In: Carey C (ed) Avian Energetics and Nutritional Ecology. Chapman and Hall, New York, pp 61–84
|
Karasov WH (2011) Digestive physiology: a view from molecules to ecosystem. Am J Physiol 301:R276–R284
|
MacKinnon J, Phillipps K (2000) A Field Guide to the Birds of China. Oxford University Press, London
|
Marsh RL, Dawson WR (1989) Avian Adjustments to Cold. In: Wang LCH (ed) Advances in Comparative and Environmental Physiology 4: Animal Adaptation to Cold. Springer, New York, pp 205–253
|
Ni XY, Lin L, Zhou FF, Wang XH, Liu JS (2011) Effect of photoperiod on body mass, organ masses and energy metabolism in Chinese bulbul (Pycnonotus sinensis). Acta Ecol Sin 31:1703–1713
|
Pang BZ (1981) Diet habit of Pycnonotus sinensis. Chin J Zool 4:75–76
|
Peng HY, Wen QH, Huang J, Huang YX (2008) The study of spring diet habit of three species of pycnonotidae. Sichuang J Zool 27:99–101
|
Sibley RM (1981) Strategies in Digestion and Defecation. In: Townsend CR, Calow P (eds) Physiological Ecology: an Evolutionary Approach to Resource Use. Blackwell, Oxford, pp 109–139
|
Starck JM (1999b) Structural flexibility of the gastro–intestinal tract of vertebrates–implications for evolutionary morphology. Zool Anz 238:87–101
|
Swanson DL (2010) Seasonal Metabolic Variation in Birds: Functional and Mechanistic Correlates. In: Thompson CF (ed) Current Ornithology. Springer, Berlin, pp 75–129
|
Zheng GM, Zhang CZ (2002) Birds in China. China Forestry Publishing House, Beijing
|
Model | CONSTANT | SPVAR | EXVAR | BOTHVAR |
Likelihood | 312.81 | 312.69 | 312.81 | 312.63 |
AIC | -621.62 | -619.38 | -619.61 | -617.27 |
Parameters | ||||
λ0 | 0.174 | 0.193 | 0.1 85 | 0.186 |
μ0 | 0.064 | 0.014 | 0.011 | 0.001 |
K | - | 0.001 | - | 0.001 |
Z | - | 1.003 | 0.078 | |
The best-fitted model is marked in boldface. |