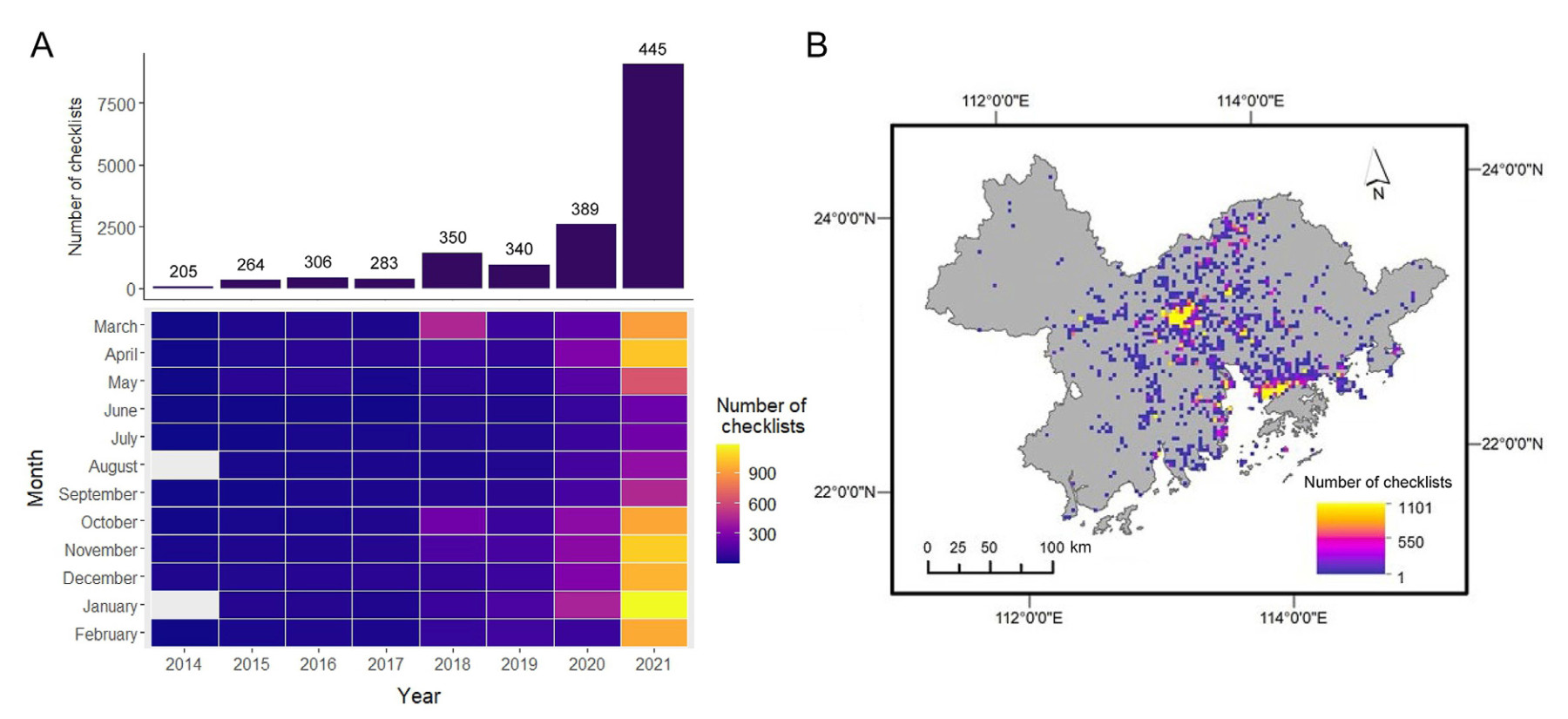
Citation: | Xilin Huang, Yihong Wang, Yang Liu, Lyu Bing Zhang. 2023: Data reliability of the emerging citizen science in the Greater Bay Area of China. Avian Research, 14(1): 100117. DOI: 10.1016/j.avrs.2023.100117 |
The potential of citizen science projects in research has been increasingly acknowledged, but the substantial engagement of these projects is restricted by the quality of citizen science data. Based on the largest emerging citizen science project in the country—Birdreport Online Database (BOD), we examined the biases of birdwatching data from the Greater Bay Area of China. The results show that the sampling effort is disparate among land cover types due to contributors' preference towards urban and suburban areas, indicating the environment suitable for species existence could be underrepresented in the BOD data. We tested the contributors' skill of species identification via a questionnaire targeting the citizen birders in the Greater Bay Area. The questionnaire show that most citizen birdwatchers could correctly identify the common species widely distributed in Southern China and the less common species with conspicuous morphological characteristics, while failed to identify the species from Alaudidae, Caprimulgidae, Emberizidae, Phylloscopidae, Scolopacidae and Scotocercidae. With a study example, we demonstrate that spatially clustered birdwatching visits can cause underestimation of species richness in insufficiently sampled areas; and the result of species richness mapping is sensitive to the contributors' skill of identifying bird species. Our results address how avian research can be influenced by the reliability of citizen science data in a region of generally high accessibility, and highlight the necessity of pre-analysis scrutiny on data reliability regarding to research aims at all spatial and temporal scales. To improve the data quality, we suggest to equip the data collection frame of BOD with a flexible filter for bird abundance, and questionnaires that collect information related to contributors' bird identification skill. Statistic modelling approaches are encouraged to apply for correcting the bias of sampling effort.
Citizen science is an important supplement to structured ecological monitoring for its capacity of acquiring massive data at fine resolution (Hochachka et al., 2012). As a recreational activity with a long history and a great many participants, birdwatching is providing tremendous data on occurrence, abundance, and habitat use of bird species in the form of citizen science projects (Sullivan et al., 2014; Wood et al., 2011). In the past 10 years, these projects (such as eBird, iNaturalist, Christmas Bird Count) have been increasingly involving in the fields of avian research including population dynamics (Horns et al., 2018), species distribution (Zulian et al., 2021), and changes of avian community structure (Lee et al., 2021). By building scientific knowledge, they have improved conservation efforts and have supported conservation-related policies, plans and actions in many regions of the world (Johnston et al., 2015; Chandler et al., 2017; McKinley et al., 2017; Young et al., 2019).
However, the quality of citizen science data has always been a challenge for researchers who intend to answer scientific questions with them (Lukyanenko et al., 2016). To obtain as many data as possible, citizen science projects usually set fewer requirements for data collection than structured surveys, and consequently generate datasets containing a larger amount of noises (Sullivan et al., 2014). Data quality of citizen birdwatching projects is susceptible to sampling effort, detection probability and bird identification skills of observers, which can be highly variable among the surveys (Farmer et al., 2014). Data quality of these projects is also affected by non-random distribution of citizen surveys across time, space and species (Gardiner et al., 2012; Kelling et al., 2015a; Swanson et al., 2016). To reduce the influence of these issues, the frame of data collection was suggested to focus on two aspects: (1) excluding records of the species that were not present (pseudo positives) through incorporating filters into data collection and review process (Silvertown, 2009); (2) reducing the biases introduced by unintentionally ignoring species that were present (pseudo negatives) through statistic estimation (Fitzpatrick et al., 2009). Recent studies additionally emphasized that the process of data cleaning should be tailored to individual projects to ensure an efficient reduction of data noises (Robinson et al., 2021; Shen et al., 2023), especially for those projects lacking a mature framework of data collection and review in the regions with a short history of birdwatching (Devictor et al., 2010).
In China, birdwatching is an emerging activity that started about 20 years ago, and the number of citizens involved has grown rapidly since then (Cheng et al., 2013; Ma et al., 2013). Aiming at data publicly sharing, the Birdreport Online Darabase (BOD) was launched by a non-government organization in 2014, and has compiled the country's most comprehensive database of citizen science (China Birdwatching Association, 2023). This Chinese online website stores bird records uploaded by citizen scientists, birders and volunteers, and provides open access to the records for all users (China Birdwatching Association, 2023). A few years after its launch, BOD started to provide data of bird species distribution for avian research and conservation at local and national scale (Hu et al., 2020; Sun et al., 2022). To reduce pseudo positive records, BOD sets a data filter based on the spatial and temporal range of the 1,501 species present in China (China Birdwatching Association, 2023), which was developed by the most knowledgeable ornithologists in the country. In the BOD dataset, the records inconsistent with the temporal or spatial range of the species are marked as “unexpected”, but pseudo positive records matching the filter cannot be identified.
In this study, we assessed the reliability of BOD data in terms of species identification and species richness estimation, using the BOD checklists documented in nine municipalities in the Guangdong–Hong Kong–Macao Greater Bay Area (the Greater Bay Area hereafter), China. Guided by the country's key strategy of innovation and opening-up, the area develops at an unprecedented speed and is witnessing rapid changes of human activities (Hui et al., 2020). Therefore, it is timely to acknowledge the potential of citizen science in facilitating conservation planning and policies. By reviewing and analyzing the BOD data, our study evaluated the bias introduced by non-randomly distributed sampling efforts of citizen surveys. As questionnaires recently become suitable tools for exploring topics related to public contribution in ecological projects (White et al., 2005; Wang et al., 2022), we assessed bird identification skills of BOD contributors via such an approach, and examined the effect of species misidentification using the spatial pattern of species richness as an example. Our study additionally discussed the potential applications and caveats of the current BOD data in avian research and suggested necessary approaches to ensure the data quality, which is expected to provide insights for a wide range of research with emerging citizen science projects.
The study is conducted with the BOD checklists documented from January 2014 to February 2022 in the nine municipalities of the Greater Bay Area located in Guangdong Province, including Guangzhou, Shenzhen, Zhuhai, Foshan, Huizhou, Dongguan, Zhongshan, Jiangmen and Zhaoqing. This area provides critical stopover and wintering sites for the birds migrating along the world's most intensively used migratory route—the East Asia–Western Australia Flyway (Kong, 2020). The Greater Bay Area is a key cooperation area established based on the agreement signed in 2017 by the three governments (National Development and Reform Commission et al., 2017), and the population of the Greater Bay Area is estimated to exceed 100 million with intensifying interactions between human and wildlife in the next 10 to 20 years (Niu et al., 2021).
During the period we examined 22,860 checklists documented in the study area. The distribution pattern of citizen surveys was examined to reveal sampling non-randomness of the BOD data. In the BOD dataset, one bird checklist contains the result of a single survey conducted by one observer, or by multiple observers conducting the same group birdwatching activity. The data collection framework requires users to fill the common name and quantity (optional) of observed species, together with the date, duration, location description and geographic coordinates of the survey. Because members of the same group activity can use different user IDs to generate checklists with identical survey results, we removed 3,984 checklists with the same coordinates and start time. Considering that most birders conducted the observations at point locations or along routes between 1 and 3 km (Callaghan et al., 2019), we mapped the locations of 18,876 citizen surveys in the Greater Bay Area according to their coordinates, and summed the number of surveys within each 3 km × 3 km grid in ArcMap 10.8 (Esri, 2020), where the surveys located on the border of two grids were counted only once. The temporal pattern was examined by summing the number of surveys conducted in each month of the study period according to the start time of the survey. With the number of checklists uploaded monthly, the seasonal average effort of citizen surveys was then calculated for March to May (spring), June to August (summer), September to November (autumn) and December to February (winter).
We evaluated the reliability of BOD data based on an online questionnaire distributed among the birders in the Greater Bay Area. With a focus on the BOD contributors, the questionnaire tested respondents' capability of identifying bird species correctly, and collected respondents' birdwatching background information that may affect their bird identification skills. The bird test was composed of high-resolution photos of 48 bird species with three choices of species common names (Chinese) provided for each. The species for test were selected from the 482 species documented by BOD users in the Greater Bay Area since 2014, through a process involved by three knowledgeable experts who have been constantly birdwatching in Southern China for more than 10 years. First, each expert independently classified the 482 species into difficulty levels of “clear” and “obscure”, according to his or her opinion on if the species can be correctly identified by most birders (yes–“clear”; no–“obscure”). The experts then reviewed the species with inconsistent difficulty levels together and reached an agreement after discussion. Based on the classification agreed by all, 20 and 28 species were randomly selected from “clear” and “obscure” subset, respectively, and photos that show the morphological traits for identification were accordingly provided for each species by the experts and volunteers. Additionally, the experts provided common names of another two morphologically similar species distributed in the Greater Bay Area to construct the multiple-choice test. With the questionnaire, we collected the information relevant to contributors' birdwatching experience including respondents' age, annual frequency of birdwatching, the time engaging in birdwatching, residential area, as well as whether the respondent is a user of BOD. We restricted the time of responding to the questionnaire within 15 min for each social network ID, and discarded repeated responses from the same ID. We distributed the questionnaire through the social network majorly used by birders in Southern China, and stopped collecting the questionnaire when the number of new responses was below five per day. The full version of questionnaire is provided as the Appendix A.
After retrieving all the responses to the questionnaire, we calculated the probability of misidentification of species i (Pmi) following:
Pmi=nmi╱nti |
where nmi was the number of misidentification and nti was the number of total responses to species i. Mixed-effect models were then constructed to examine the effects of a series of potential predictor variables (xn) on the probability of misidentification (Pm) including random effects introduced by variables r1 and r2, following the syntax in R language:
Pm∼ x1+x2+…xn+(1|r1)+(1|r2) |
We assumed that a majority of birders in China identified bird species by morphological clues. Since these clues are also used for developing bird taxonomy, we included the family of species as a potential predictor of Pm. Life-history traits associated with the pattern and color of feathers, such as age-class and nuptial plumage, were also included. Adult body mass and primary lifestyle were included as predictors, given that the size and life form of birds might influence the correctness of identification. The data of above variables were extracted using species name from the AVONET dataset of avian morphological, ecological and geographical traits (Tobias et al., 2022) and the website of Birds of the World (The Cornell Laboratory of Ornithology, 2022). Random effects were introduced by the heterogeneity among species (r1) and whether the respondent was a BOD user (r2). See the detailed information of all variables in Appendix B: Table S1. Based on the results of the mixed-effect model, the experts re-evaluated the difficulty levels of bird identification.
We further illustrated the biases introduced by non-random sampling and species misidentification by mapping the species records from all checklists according to the observation coordinates. Given the common survey range of a single birdwatching activity, we resampled the study area with 3 × 3 km2 grid and counted the number of “clear” and “obscure” records of species for each grid. The records of the same species within the same grid were considered as spatially replicated and counted once. To show how the number of observed species changes with sampling effort, the count of species was plotted against the count of surveys (checklists) and fitted with logarithm regression for species of different difficulty levels of identification. We additionally compared the sampling effort of the six land cover types in the Greater Bay Area by calculating the ratio of the sample to the total area for each type. The spatial analysis was conducted with Arcmap 10.8 (Esri, 2020).
The BOD data showed the citizen survey efforts across time and space were highly clustered in the Greater Bay Area over the past eight years. After BOD's launch, the annual number of checklists in the Greater Bay Area had two dramatic increases. The first one was in 2018 which almost tripled the number of checklists in the previous year, and the second was in 2021 with an annual increasing rate of 2.48, accounting for 59.47% of all the checklists uploaded to BOD since 2014 (Fig. 1A). The number of checklists also showed inter-seasonal variations with generally more checklists recorded during October to April than in other times of the year (Fig. 1A). After removing the replicated checklists, we grouped the start time of survey by season, and found the number of checklists in summer (189.13 ± 284.12, n = 8) was lower than in other seasons (PSpring–Summer = 0.0078, PSummer–Autumn = 0.0078, PSummer–Winter = 0.0078, while no difference was shown among spring (563.5 ± 861.70, n = 8), autumn (535.13 ± 825.56, n = 8) and winter (617.75 ± 1045.30, n = 8) (paired Wilcoxon test, P > 0.05). The spatial coverage of citizen bird surveys, measured with the number of sample grids with BOD checklist(s), was 16.35% for the whole Greater Bay Area. The survey locations were spatial auto-correlated (Moran's I = 0.3397, z-score: 58.5856, P < 0.0001), 83.56% of which were concentrated in the two largest municipalities–Guangzhou and Shenzhen, while the survey effort was relatively low in the northwestern (Zhaoqing), southwestern (Jiangmen and Zhongshan) and eastern (Huizhou) Greater Bay Area (Fig. 1B). Among the six major land cover types in the Greater Bay Area, we found the survey coverage was skewed towards the construction lands (38.59% of 6,628.39 km2) including cities, villages roads and industrial lands. The survey coverage was the lowest in the evergreen coniferous forest (7.07% of 6,094.59 km2), followed by the farmlands (12% of 4,456.18 km2) and evergreen broadleaf forests (12.09% of 30,149 km2).
We retrieved the questionnaire from 564 respondents in 9 days, 51.77% of which are BOD contributors. Nearly a half (47.26%) of the respondents have a birdwatching experience of 3–5 years, and 67.47% engaged in the birdwatching activities more than 10 times per year. Over half of the respondents (67.12%) were aged between 18 and 45 years old. The respondents' mean score of the bird identification test was 69.18 ± 14.66 (n = 564), with a median of 68. We found that BOD contributors scored higher on the test than non-contributors (F1, 359 = 30.98, P < 0.001), and the scores were higher for the respondents with a birdwatching experience over 5 years (F2, 561 = 46.59, P < 0.001). Respondent age showed no correlation with their test scores (F2, 561 = 1.60, P = 0.1895). Additionally, higher scores were shown for the respondents who engage in birdwatching activities more than 10 times per year (F3, 560 = 66.24, P < 0.001).
We calculated the probability of misidentification for all 48 species in the test, and the detailed results were shown in Appendix B: Table S1. The probability of misidentification of 18 species was less than 0.1, 72% of which were classified as “clear” in the expert evaluation. Most of these are the common species widely distributed in Southern China, or less-common species with conspicuous morphological characteristics, such as Pycnonotus jocosus, Turdus mandarinus and Platalea minor. Adult body mass and the taxonomic family of the species together explained 62.61% of the difference among the probability of misidentification of the 48 species (Table 1). The respondents were more likely to misidentify the species from Alaudidae, Caprimulgidae, Emberizidae, Phylloscopidae, Scolopacidae and Scotocercidae (Fig. 2) which show high level of morphological resemblance, especially the ones of larger sizes from these families. Based on the questionnaire results, the experts adjusted the difficulty level of identification of 16 species and generated the final classification (see Appendix B: Table S1).
Moderator | Estimate | Standard error | dfa | t | Pb |
(Intercept)(Accipitridae) | −2.5186 | 1.8757 | 12.2471 | −1.343 | 0.2037 |
Body mass | 0.4712 | 0.2138 | 12 | 2.204 | 0.0478* |
Sexual dimorphism | −0.5581 | 0.4864 | 12 | −1.147 | 0.2736 |
Age polymorphism | 0.4006 | 0.5081 | 12 | 0.788 | 0.4458 |
Nuptial plumage | 0.2069 | 0.4084 | 12 | 0.507 | 0.6215 |
Family | |||||
Alaudidae | 3.6844 | 1.3970 | 12 | 2.637 | 0.0217* |
Alcedinidae | 0.1275 | 0.9839 | 12 | 0.130 | 0.8990 |
Ardeidae | 0.6721 | 1.0604 | 12 | 0.634 | 0.5381 |
Campephagidae | 2.7577 | 1.3693 | 12 | 2.014 | 0.0670. |
Caprimulgidae | 2.3122 | 1.1651 | 12 | 1.985 | 0.0705. |
Charadriidae | 1.1248 | 1.4113 | 12 | 0.797 | 0.4409 |
Cisticolidae | 1.9201 | 1.2615 | 12 | 1.522 | 0.1539 |
Corvidae | −0.8623 | 0.8889 | 12 | −0.970 | 0.3512 |
Cuculidae | 1.2053 | 1.2031 | 12 | 1.002 | 0.3362 |
Emberizidae | 3.8831 | 1.3942 | 12 | 2.785 | 0.0165* |
Hirundinidae | 0.7066 | 1.4646 | 12 | 0.482 | 0.6381 |
Leiothrichidae | 0.7579 | 1.1589 | 12 | 0.654 | 0.5255 |
Locustellidae | −0.8415 | 1.1407 | 12 | −0.738 | 0.4749 |
Motacillidae | 1.8589 | 1.3233 | 12 | 1.405 | 0.1854 |
Muscicapidae | 1.3381 | 0.9548 | 12 | 1.401 | 0.1864 |
Nectariniidae | 1.9255 | 1.3421 | 12 | 1.435 | 0.1769 |
Paridae | 0.8602 | 1.1812 | 12 | 0.728 | 0.4804 |
Phylloscopidae | 3.0610 | 1.1482 | 12 | 2.666 | 0.0206* |
Podicipedidae | −1.2934 | 1.3226 | 12 | −0.978 | 0.3474 |
Pycnonotidae | −0.0497 | 1.0658 | 12 | −0.047 | 0.9636 |
Rallidae | 0.4782 | 1.2388 | 12 | 0.386 | 0.7063 |
Scolopacidae | 1.9426 | 1.1604 | 12 | 1.674 | 0.1200 |
Scotocercidae | 0.8191 | 1.0353 | 12 | 0.791 | 0.4442 |
Sturnidae | 1.1735 | 1.2168 | 12 | 0.964 | 0.3539 |
Threskiornithidae | −1.0967 | 1.1969 | 12 | −0.916 | 0.3775 |
Timaliidae | 0.8740 | 1.3091 | 12 | 0.668 | 0.5170 |
Turdidae | −1.8864 | 0.8524 | 12 | −2.213 | 0.0470* |
Zosteropidae | 1.1216 | 1.2957 | 12 | 0.866 | 0.4037 |
Lifestyle | |||||
Generalistc | 1.2242 | 0.9356 | 12 | 1.308 | 0.2152 |
Insessoriald | −0.5451 | 0.9522 | 12 | −0.572 | 0.5776 |
Terrestriale | −1.3721 | 1.0804 | 12 | −1.270 | 0.2282 |
Random effects | Variance | SD | |||
Species (Intercept) | 0.37587 | 0.6131 | |||
User (Intercept) | 0.07654 | 0.2767 | |||
Residual | 0.04711 | 0.2171 | |||
a df: degree of freedom. Maximum number of logically independent values, which are values that have the freedom to vary, in the data sample. b Significance level: “*” marks a P-value < 0.05, and “.” marks a P-value < 0.1. c Generalist: species has no primary lifestyle because it spends time in different lifestyle classes. d Insessorial: species spends much of the time perching above the ground, either in branches of trees and other vegetation (i.e., arboreal), or on other raised substrates including rocks, buildings, posts, and wires. e Terrestrial: species spends majority of its time on the ground, where it obtains food while either walking or hopping (note this includes species that also wade in water with their body raised above the water. |
Our example illustrates that bird species appear to concentrate in northeastern mountainous areas and urban areas of Guangzhou and Shenzhen as we mapped species richness using the original BOD data (Fig. 3A). We estimated number of species at the same sampling effort, and find the mountainous areas in the eastern and northeastern Greater Bay Area are of high richness of “clear” species (Fig. 3B). The estimated richness of all documented species was the highest in the farmland adjacent to the construction land in the central, southeastern and southwestern of the Greater Bay Area (Fig. 3C), and a large proportion of the checklist species from these areas (69.80%) were “obscure” species. The checklists from wetlands and grassland also showed a high proportion of “obscure” species (56.54% and 56.32%, respectively), comparing with the other three land cover types.
For the Greater Bay Area, over 92.10% of the checklists were documented in the last four years after the BOD platform was popularized among a significant number of citizen birders. The first soar of checklists was largely attributed to the release of BOD mobile application in 2018, which facilitated a rapid increase in the number of contributors by enabling the timely documentation of observed bird species with cellphones. The other dramatic increase of checklists might be associated with the activity–“Reservation Big Year” promoted by BOD in 2021, which encourages the contributors to visit the same location repeatedly (a reservation) to increase the number of observed species in their big-year lists. Nevertheless, the annual number of documented species did not increase much despite the boom of checklists (Fig. 1A), indicating a decrease of new species along with the growing survey effort. The BOD contributors also showed a preference towards spring and autumn when the probability of spotting an uncommon species was higher due to bird migration. Comparatively, birdwatching trips were not as cost-effective in summer of Southern China, because of a lower chance of documenting uncommon species and the high average temperature (~30.4 ℃) (Ruan et al., 2022).
The spatial distribution of surveys indicated that the birdwatching activities biased towards high level of urbanization in the Greater Bay Area. The bias can be attributed to the composition of BOD contributors and location accessibility. As a recreational activity, well-developed birdwatching is usually associated with a high economic level of the society (Hvenegaard, 2002; Zhang et al., 2022). Guangzhou and Shenzhen are the two of the most populated and economically vigorous municipalities in the Greater Bay Area, where the number of residents participating in birdwatching is also much higher than of the others. In these two areas, the resident birders were frequently birdwatching in the places easy to access such as urban green space and suburban agricultural lands and reservoirs, and consequently had a larger contribution to the BOD data. As such, the further an area is from Guangzhou and Shenzhen, the less citizen survey effort it received. In our example, we controlled such a bias by estimating the number of species at the same sampling effort (n = 250, Appendix B: Fig. S1), and find that the hotspots of species richness shifted to the farmlands and forests from the economic center of the Greater Bay Area (Fig. 3).
Misidentification is another issue that affects the quality of BOD data. From the results of bird identification test, we concluded that most respondents were “backyard birders” who were reliable in identifying the most common species of their residential region. They were also performed well with species that have outstanding morphological characteristics, such as distinctively shaped or colored body parts and bright plumage colors. As to the other species, the misidentification probability was higher. These species are usually from a family or genus where species are morphologically similar but the cues for distinguishing one from another are not noticeable. The cues can be subtle differences in morphology, or distinctive behavioral or vocal characteristics. Identification of the cues often requires sufficient birdwatching experience which was not available for the most of respondents who have engaged in birdwatching for less than 10 years. Inexperienced respondents can be familiar with none or some of the obscure species and their skills vary among species due to different birdwatching experience. Therefore, large variances were shown for the misidentification probability of the obscured species in our questionnaire.
Our application example shows that the result of species richness mapping was sensitive to the contributors' skills in bird identification, and could be affected by non-random sampling effort, and the difference of detection probability among land cover types. Due to misidentification, one species can be recorded as one or more different species in the checklists, and consequently inflate the total number of species documented in the region. The inconsistency shown in Fig. 3 was attributed to the higher proportion of “obscure” species in farmland, grassland and wetland. The three land cover types are all open and of low heterogeneity, where observers would have a wider vision than in the forests and consequently a higher detection probability of birds. These land cover types are also the major habitats of obscure species from Alaudidae, Emberizidae and Scolopacidae which challenge many observers' skills of bird identification.
The BOD data is more likely to receive pseudo positives and negative inputs from inexperienced contributors due to their poor skills in bird observation and identification. Without assessing contributors' bird identification skills, it is difficult to distinguish the reliable information from the noises in the data, and researchers who attempt to use the data need to be aware of the errors associated with such an issue. To overcome this, the assessment of contributors' skills is encouraged to be incorporated either into the data collection frame of BOD or as a separated process in the research, to evaluate the bias associated with the observation process (Johnston et al., 2017). The assessment can be organized with an expertise-scoring system that describes inter-observer variation in species identification and detectability (Kelling et al., 2015b), based on the information related to contributors' birding experience, such as annual birding days and the length of life list, and species identification tests. Vocal and behavioral information of birds that were not provided in our questionnaire are encouraged to be included in future species identification tests as relevant recourses accumulates.
Globally, the sampling effort on biodiversity made through human is positively related with GDP per capita and highly skewed towards the vicinity of transportation routes, and these biases are often exacerbated in the inputs of citizen scientists (Hughes et al., 2021). The same pattern was identified from the citizen birdwatching observations in the Greater Bay Area, where the sampling made by BOD users is generally poor in the less-developed and remote areas (Fig. 1B). Such biases contributed to the disparity of sampling effort among land cover types, indicating that the environment suitable for species existence could be underrepresented in the BOD data within the study area. Although statistical approaches such as species distribution models are a popular way to overcome biases and reconstruct species ranges, lacking records from intact habitat prevents the estimation of accurate biodiversity patterns, which can be especially restricting at finer scales (Lira-Noriega et al., 2007; Qiao et al., 2017).
The performance of BOD data was also poor in indicating the dynamics of bird populations, due to the low reliability of abundance data. In the data collection frame of BOD, abundance of an individual species is a required filed with a defaulted minimum value of one. The setting prevents us distinguishing the situation where contributors cannot provide the abundance data from those where one individual is actually observed. Comparatively, eBird, the world's largest biodiversity-related citizen science project, builds a more flexible frame for collecting the data of bird abundance. When starting an eBird checklist, contributors are required to choose the observation type (traveling, stationary, historical, incidental and other) which is associated with a specific data frame. The defaulted value of abundance is “null” to separate the case of no data from the rest, and a maximum threshold (estimated with historical data of the species) is set for abundance to identify the unexpectedly large inputs. Contributors of these inputs will be contacted for supporting information of their data. eBird additionally asks the contributors whether their checklists are complete ones that include all the species that the users observe and identify at their best. We consider that BOD could improve the quality of abundance data by developing effective filters associated with data availability and threshold. On the other hand, the data quality can be also improved by providing guidance on bird counting for contributors.
The impact of misidentification and sampling bias on BOD data have been demonstrated, which are important issues faced by citizen science projects (Kelling et al., 2019). Scientific applications of the BOD data are closely associated to the data requirements defined by the aims of research. Clear aims will enable targeted assessment of data quality, which is a critical first step to avoid data misuse (Orr et al., 2021). For those data of useable form, the assessment will also contribute to the development of effective data cleaning processes to eliminate bias and error. Therefore, we suggest prudence regarding research scales subject to the sampling bias, and prioritization of research targets that can be fulfilled with the data of high reliability. For example, to reduce the uncertainties associated with pseudo negative records caused by species misidentification, research may focus on observations of the species that can be identified by most citizen birders based on the evaluation of their bird identification skills. Such practices limit the knowledge of species diversity, but can still reveal the variations in phenology or range of common, human-associated or charismatic bird species.
Although the highly skewed sampling effort restricts the application of BOD data in revealing bird distribution, we consider the data has a great potential in supporting research that focus on the occurrence of urban and sub-urban bird species. On the other hand, recognizing the sampling bias of BOD would also help allocate survey efforts towards the biomes and areas poorly-investigated by citizens. Recent studies have increasingly applied statistic modelling to reduce the bias of sampling effort, which provides insights for estimating species distribution using citizen science data. In these studies, sampling effort was measured as the exposure of a given location to citizens, which was predicted by the visibility, accessibility (Zhu et al., 2015), or geographic features of the location (Tang et al., 2021).
With the BOD data from the Greater Bay Area of China, our results described the influence of reliability of citizen science data on avian research in a region with high accessibility and urbanization level. We highlight the necessity of pre-analysis scrutiny on data reliability regarding to research aims at all spatial and temporal scales. Despite the application limitations, the BOD is an important supplement to the structured bird surveys at local scales carried out by research and management institutions. It also helps to optimize the sampling effort by supporting effective prioritization of sampling areas based on a stratified method considering habitat heterogeneity. We see a great potential of the BOD in becoming one of the major contributors of bird occurrence data in China, and a large space of improvement in its current frame of data collection. Overcoming these shortfalls will increase the quality of public data and reduce biased research implications accordingly.
L.B. Zhang designed the study, X. Huang and Y. Wang performed the analysis, L.B. Zhang and X. Huang wrote the manuscript. All authors contributed to the revison of the manuscript.
The authors declare that they have no competing of interests.
We appreciate China Birdwatching Association for providing the birdwatching data of the Greater Bay Area, China. We would also thank all the skillful birders who contributed to the construction of the bird identification test.
Supplementary data to this article can be found online at https://doi.org/10.1016/j.avrs.2023.100117.
Moderator | Estimate | Standard error | dfa | t | Pb |
(Intercept)(Accipitridae) | −2.5186 | 1.8757 | 12.2471 | −1.343 | 0.2037 |
Body mass | 0.4712 | 0.2138 | 12 | 2.204 | 0.0478* |
Sexual dimorphism | −0.5581 | 0.4864 | 12 | −1.147 | 0.2736 |
Age polymorphism | 0.4006 | 0.5081 | 12 | 0.788 | 0.4458 |
Nuptial plumage | 0.2069 | 0.4084 | 12 | 0.507 | 0.6215 |
Family | |||||
Alaudidae | 3.6844 | 1.3970 | 12 | 2.637 | 0.0217* |
Alcedinidae | 0.1275 | 0.9839 | 12 | 0.130 | 0.8990 |
Ardeidae | 0.6721 | 1.0604 | 12 | 0.634 | 0.5381 |
Campephagidae | 2.7577 | 1.3693 | 12 | 2.014 | 0.0670. |
Caprimulgidae | 2.3122 | 1.1651 | 12 | 1.985 | 0.0705. |
Charadriidae | 1.1248 | 1.4113 | 12 | 0.797 | 0.4409 |
Cisticolidae | 1.9201 | 1.2615 | 12 | 1.522 | 0.1539 |
Corvidae | −0.8623 | 0.8889 | 12 | −0.970 | 0.3512 |
Cuculidae | 1.2053 | 1.2031 | 12 | 1.002 | 0.3362 |
Emberizidae | 3.8831 | 1.3942 | 12 | 2.785 | 0.0165* |
Hirundinidae | 0.7066 | 1.4646 | 12 | 0.482 | 0.6381 |
Leiothrichidae | 0.7579 | 1.1589 | 12 | 0.654 | 0.5255 |
Locustellidae | −0.8415 | 1.1407 | 12 | −0.738 | 0.4749 |
Motacillidae | 1.8589 | 1.3233 | 12 | 1.405 | 0.1854 |
Muscicapidae | 1.3381 | 0.9548 | 12 | 1.401 | 0.1864 |
Nectariniidae | 1.9255 | 1.3421 | 12 | 1.435 | 0.1769 |
Paridae | 0.8602 | 1.1812 | 12 | 0.728 | 0.4804 |
Phylloscopidae | 3.0610 | 1.1482 | 12 | 2.666 | 0.0206* |
Podicipedidae | −1.2934 | 1.3226 | 12 | −0.978 | 0.3474 |
Pycnonotidae | −0.0497 | 1.0658 | 12 | −0.047 | 0.9636 |
Rallidae | 0.4782 | 1.2388 | 12 | 0.386 | 0.7063 |
Scolopacidae | 1.9426 | 1.1604 | 12 | 1.674 | 0.1200 |
Scotocercidae | 0.8191 | 1.0353 | 12 | 0.791 | 0.4442 |
Sturnidae | 1.1735 | 1.2168 | 12 | 0.964 | 0.3539 |
Threskiornithidae | −1.0967 | 1.1969 | 12 | −0.916 | 0.3775 |
Timaliidae | 0.8740 | 1.3091 | 12 | 0.668 | 0.5170 |
Turdidae | −1.8864 | 0.8524 | 12 | −2.213 | 0.0470* |
Zosteropidae | 1.1216 | 1.2957 | 12 | 0.866 | 0.4037 |
Lifestyle | |||||
Generalistc | 1.2242 | 0.9356 | 12 | 1.308 | 0.2152 |
Insessoriald | −0.5451 | 0.9522 | 12 | −0.572 | 0.5776 |
Terrestriale | −1.3721 | 1.0804 | 12 | −1.270 | 0.2282 |
Random effects | Variance | SD | |||
Species (Intercept) | 0.37587 | 0.6131 | |||
User (Intercept) | 0.07654 | 0.2767 | |||
Residual | 0.04711 | 0.2171 | |||
a df: degree of freedom. Maximum number of logically independent values, which are values that have the freedom to vary, in the data sample. b Significance level: “*” marks a P-value < 0.05, and “.” marks a P-value < 0.1. c Generalist: species has no primary lifestyle because it spends time in different lifestyle classes. d Insessorial: species spends much of the time perching above the ground, either in branches of trees and other vegetation (i.e., arboreal), or on other raised substrates including rocks, buildings, posts, and wires. e Terrestrial: species spends majority of its time on the ground, where it obtains food while either walking or hopping (note this includes species that also wade in water with their body raised above the water. |